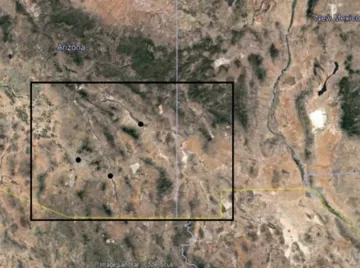
Study area in Southeastern Arizona and Southwestern New Mexico, bound by the black box. Dots are fixed-point temperature locations.
Snowfall in the Madrean Sky Islands is a crucial process sustaining and recharging the groundwater supply of the deserts of Southeastern Arizona, but a lack of in situ observations and poor forecasting of events hinder hydrological modeling of the recharge process. Snowfall forecast is routinely overpredicted due to issues in predicting snow-liquid ratios (SLR) ahead of major events. Current study is testing multiple machine learning methods for dynamic SLR prediction for the Sky Islands region. Input parameters were chosen based off variables found by previous studies to have a significant relationship with SLR, with a focus on the lower-mid levels of the troposphere. Initial tests of the network are highly promising accuracy-wise, and it shows significant gain in skill compared to a multiple linear regression model using the same parameters.
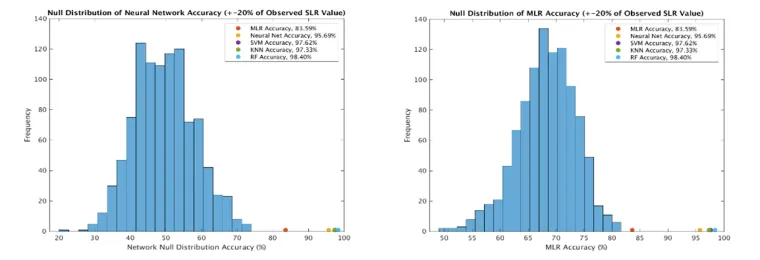
Figure: Randomized null distributions for both the MLR and neural network comparing hit rate to that of the original samples for each method. All points are significant at 99% confidence (randomized distribution is independent). Also visible is a significant gain vs the null distribution for the Neural Network compared to the MLR.